When a new type of drug or therapy is discovered, double-blind randomized controlled trials (DBRCTs) are the gold standard for evaluating them. These trials, which have been used for years, were designed to determine the true efficacy of a treatment free from patient or doctor bias, but they do not factor in the effects that patient behaviors, such as diet and lifestyle choices, can have on the tested treatment.
A recent meta-analysis of six such clinical trials, led by Caltech's Erik Snowberg, professor of economics and political science, and his colleagues Sylvain Chassang from Princeton University and Ben Seymour from Cambridge University, shows that behavior can have a serious impact on the effectiveness of a treatment—and that the currently used DBRCT procedures may not be able to assess the effects of behavior on the treatment. To solve this, the researchers propose a new trial design, called a two-by-two trial, that can account for behavior–treatment interactions.
The study was published online on June 10 in the journal PLOS ONE.
Patients behave in different ways during a trial. These behaviors can directly relate to the trial—for example, one patient who believes in the drug may religiously stick to his or her treatment regimen while someone more skeptical might skip a few doses. The behaviors may also simply relate to how the person acts in general, such as preferences in diet, exercise, and social engagement. And in the design of today's standard trials, these behaviors are not accounted for, Snowberg says.
For example, a DBRCT might randomly assign patients to one of two groups: an experimental group that receives the new treatment and a control group that does not. As the trial is double-blinded, neither the subjects nor their doctors know who falls into which group. This is intended to reduce bias from the behavior and beliefs of the patient and the doctor; the thinking is that because patients have not been specifically selected for treatment, any effects on health outcomes must be solely due to the treatment or lack of treatment.
Although the patients do not know whether they have received the treatment, they do know their probability of getting the treatment—in this case, 50 percent. And that 50 percent likelihood of getting the new treatment might not be enough to encourage a patient to change behaviors that could influence the efficacy of the drug under study, Snowberg says. For example, if you really want to lose weight and know you have a high probability—say 70 percent chance—of being in the experimental group for a new weight loss drug, you may be more likely to take the drug as directed and to make other healthy lifestyle choices that can contribute to weight loss. As a result, you might lose more weight, boosting the apparent effectiveness of the drug.
However, if you know you only have a 30 percent chance of being in the experimental group, you might be less motivated to both take the drug as directed and to make those other changes. As a result, you might lose less weight—even if you are in the treatment group—and the same drug would seem less effective.
"Most medical research just wants to know if a drug will work or not. We wanted to go a step further, designing new trials that would take into account the way people behave. As social scientists, we naturally turned to the mathematical tools of formal social science to do this," Snowberg says.
Snowberg and his colleagues found that with a new trial design, the two-by-two trial, they can tease out the effects of behavior and the interaction of behavior and treatment, as well as the effects of treatment alone. The new trial, which still randomizes treatment, also randomizes the probability of treatment—which can change a patient's behavior.
In a two-by-two trial, instead of patients first being assigned to either the experimental or control groups, they are randomly assigned to either a "high probability of treatment" group or a "low probability of treatment" group. The patients in the high probability group are then randomly assigned to either the treatment or the control group, giving them a 70 percent chance of receiving the treatment. Patients in the low probability group are also randomly assigned to treatment or control; their likelihood of receiving the treatment is 30 percent. The patients are then informed of their probability of treatment.
By randomizing both the treatment and the probability of treatment, medical researchers can quantify the effects of treatment, the effects of behavior, and the effects of the interaction between treatment and behavior. Determining each, Snowberg says, is essential for understanding the overall efficacy of treatment.
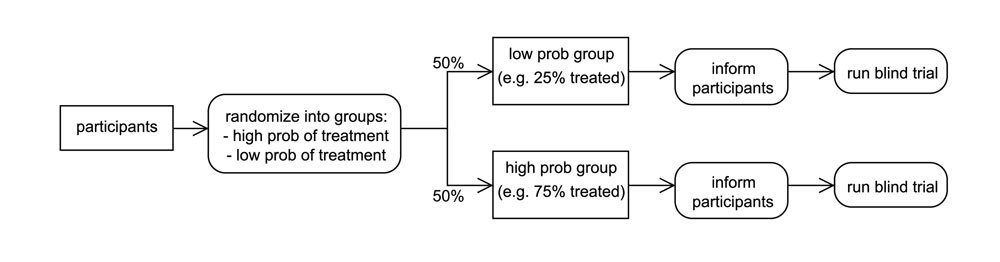
"It's a very small change to the design of the trial, but it's important. The effect of a treatment has these two constituent parts: pure treatment effect and the treatment–behavior interaction. Standard blind trials just randomize the likelihood of treatment, so you can't see this interaction. Although you can't just tell someone to randomize their behavior, we found a way that you can randomize the probability that a patient will get something that will change their behavior."
Because it is difficult to implement new trial design changes in active trials, Snowberg and his colleagues wanted to first test their idea with a meta-analysis of data from previous clinical trials. They developed a way to test this idea by coming up with a new mathematical formula that can be used to analyze DBRCT data. The formula, which teases out the health outcomes resulting from treatment alone as well as outcomes resulting from an interaction between treatment and behavior, was then used to statistically analyze six previous DBRCTs that had tested the efficacy of two antidepressant drugs, imipramine (a tricyclic antidepressant also known as Tofranil) and paroxetine (a selective serotonin reuptake inhibitor sold as Paxil).
First, the researchers wanted to see if there was evidence that patients behave differently when they have a high probability of treatment versus when they have a low probability of treatment. The previous trials recorded how many patients dropped out of the study, so this was the behavior that Snowberg and his colleagues analyzed. They found that in trials where patients happened to have a relatively high probability of treatment—near 70 percent—the dropout rate was significantly lower than in other trials with patients who had a lower probability of treatment, around 50 percent.
Although the team did not have any specific behaviors to analyze, other than dropping out of the study, they also wanted to determine if behavior in general could have added to the effect of the treatments. Using their statistical techniques, they determined that imipramine seemed to have a pure treatment effect, but no effect from the interaction between treatment and behavior—that is, the drug seemed to work fine, regardless of any behavioral differences that may have been present.
However, after their analysis, they determined that paroxetine seemed to have no effect from the treatment alone or behavior alone. However, an interaction between the treatment and behavior did effectively decrease depression. Because this was a previously performed study, the researchers cannot know which specific behavior was responsible for the interaction, but with the mathematical formula, they can tell that this behavior was necessary for the drug to be effective.
In their paper, Snowberg and his colleagues speculate how a situation like this might come about. "Maybe there is a drug, for instance, that makes people feel better in social situations, and if you're in the high probability group, then maybe you'd be more willing to go out to parties to see if the drug helps you talk to people," Snowberg explains. "Your behavior drives you to go to the party, and once you're at the party, the drug helps you feel comfortable talking to people. That would be an example of an interaction effect; you couldn't get that if people just took this drug alone at home."
Although this specific example is just speculation, Snowberg says that the team's actual results reveal that there is some behavior or set of behaviors that interact with paroxetine to effectively treat depression—and without this behavior, the drug appears to be ineffective.
"Normally what you get when you run a standard blind trial is some sort of mishmash of the treatment effect and the treatment-behavior interaction effect. But, knowing the full interaction effect is important. Our work indicates that clinical trials underestimate the efficacy of a drug where behavior matters," Snowberg says. "It may be the case that 50 percent probability isn't high enough for people to change any of their behaviors, especially if it's a really uncertain new treatment. Then it's just going to look like the drug doesn't work, and that isn't the case."
Because the meta-analysis supported the team's hypothesis—that the interaction between treatment and behavior can have an effect on health outcomes—the next step is incorporating these new ideas into an active clinical trial. Snowberg says that the best fit would be a drug trial for a condition, such as a mental health disorder or an addiction, that is known to be associated with behavior. At the very least, he says, he hopes that these results will lead the medical research community to a conversation about ways to improve the DBRCT and move past the current "gold standard."
These results are published in a paper titled "Accounting for Behavior in Treatment Effects: New Applications for Blind Trials." Cayley Bowles, a student in the UCLA/Caltech MD/PhD program, was also a coauthor on the paper. The work was supported by funding to Snowberg and Chassang from the National Science Foundation.
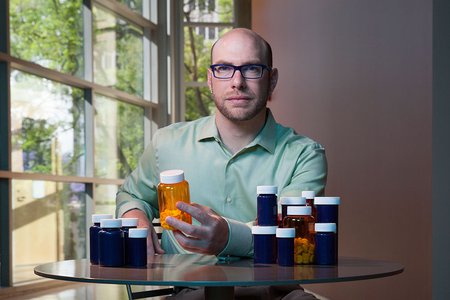
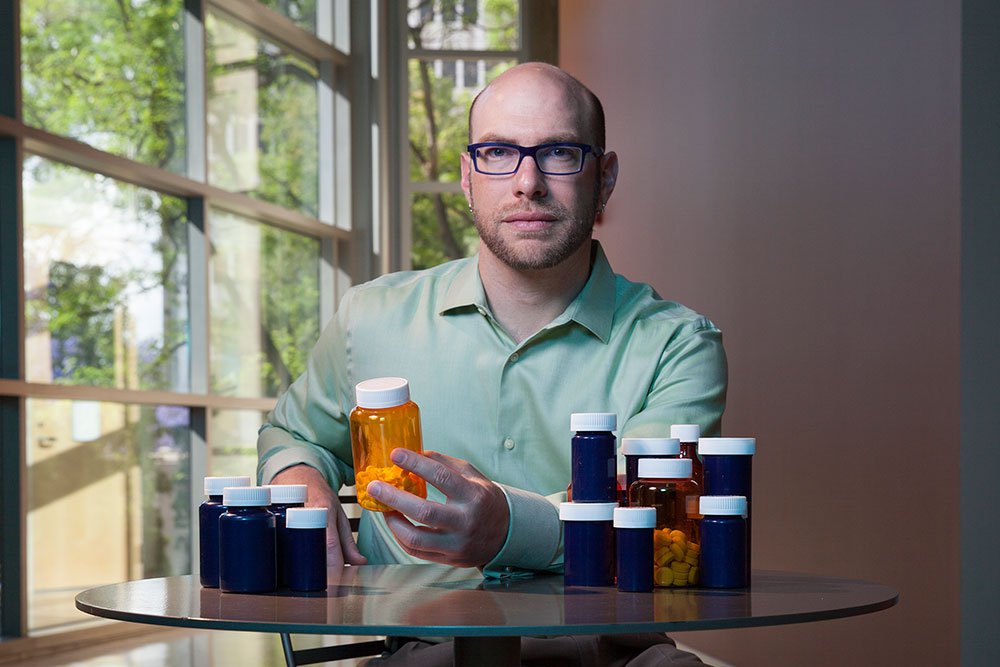