The butterfly effect—the idea that a butterfly flapping its wings in South America could change the course of a tornado in Texas—has been a popular way to illustrate the difficulty of making predictions about the behavior of chaotic systems like the weather. Because such systems are extremely sensitive to their initial conditions, even the tiniest of changes can send their behavior careening off into unexpected directions.
Scientists have become pretty good at predicting the weather, at least up to 10 days in the future. But making accurate, longer-term predictions about how climate is changing remains challenging. The ability to predict climate change is important because it can help guide decisions about insurance coverage in fire- or flood-prone areas; it can also help architects design safer and more comfortable buildings for occupants in a warmer future.
Our ability to model climate change has been hampered by the enormous amount of computing power required to simulate all facets of climate, says Tapio Schneider, the Theodore Y. Wu Professor of Environmental Science and Engineering and a senior research scientist at JPL, which Caltech manages for NASA. To make a global climate model accurate, it needs to capture small-scale processes, such as those controlling droplet formation in clouds, over the entire planet. Simulating this huge range of relevant processes is currently impossible.
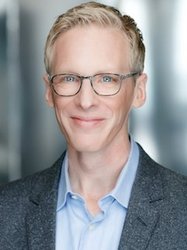
Instead, climate models calculate how temperature, winds, and ocean currents evolve on a mesh with a horizontal resolution around 50–100 kilometers. Smaller-scale processes, such as those in low-lying marine clouds that help shape the climate, are represented by rough approximations called parameterizations. These parameterizations often lack a strong foundation in both scientific principles and data.
Recently, a group of climate scientists proposed a massively scaled climate-modeling project as a solution. The project would consist of a series of centers running supercomputers that are staffed by 200–300 scientists each. The goal would be to create climate models with a resolution of 1 kilometer.
Schneider says that might prove difficult.
"Running climate models is enormously computationally expensive," Schneider says. "If you want to make the computational mesh finer by a factor of 10 in the horizontal plane, you need a thousand times the computational power."
Schneider says a project of that nature would strain our current computational abilities, and it would not be able to represent the small-scale processes necessary for accurate modeling. It would further concentrate resources among developed nations better able to fund such centers, thereby leaving out developing nations, he says.
In a paper recently published in the journal Nature Climate Change, Schneider and 13 other scientists from around the world propose to develop models with a moderately high resolution (tens of kilometers), providing a stronger scientific foundation for parameterizations of smaller-scale processes, using machine learning to fill in the gaps. This, the researchers say, will create robust models without the need for gargantuan computing centers.
"We cannot possibly resolve the marine clouds over the tropical oceans globally anytime soon because it will require a computer about a hundred billion times faster than the fastest one we have," Schneider says. "What we can do is simulate these clouds in small areas and generate data. Those data can also inform a coarser climate model."
Schneider says work on the kinds of AI tools needed to improve the coarser models is happening right now, some of it at Caltech. This technology could do for climate models what better data has done for weather models.
"Weather forecasts have become quite good over the last few decades. It's a bit of an unsung success story of science," he says. "The primary reason they've become quite good is that they use data much more extensively than they used to. In some ways, we want to achieve the same thing for climate prediction. I think that can be achieved by using AI tools to harness the extensive data about Earth we already have."
Andrew Stuart, Bren Professor of Computing and Mathematical Sciences and a co-author of the proposal, has lent his expertise to Schneider's effort, helping build the tools necessary for the project. Stuart says one set of tools aims to make climate models less computationally expensive to run.
This can be done by creating parameterizations for coarser models, which Stuart describes as "excellent sketches" of actual physical processes, such as the behavior of individual clouds. In essence, they capture the main features needed to predict the effects that small-scale processes will have on larger-scale processes in a global model simulation. Because they are simplified versions of actual events, they have to be tuned to behave like their real-life counterparts.
Even coarser models with parameterizations are expensive to run in terms of computing time and power, and the tuning process means they need to be run repeatedly until the researchers succeed in tuning them to produce reliable and realistic outputs.
"The primary computational challenge is to perform the tuning with the smallest number of costly model evaluations," Stuart says. "We do that by building on ideas that go back to 1960 and an engineer named Rudolf Kálmán; the ideas have now evolved into what are called ensemble Kalman filters. They allow us to make predictions and calibration with the minimal number of evaluations. They are used in weather forecasting, and we've repurposed them."
The second set of tools Stuart has worked on in the climate arena fall under the umbrella of what might be popularly referred to as A.I., and more specifically, machine learning. He says machine learning can help quantify some of the uncertainty inherent in climate modeling and generate new data at scales that are hard to measure.
"The models have an enormous amount of uncertainty in them," Stuart says. "This is where machine learning enters."
The machine learning used by the team has similarities to image generators like OpenAI's DALL-E. In the case of DALL-E, the machine-learning algorithm is trained on a huge set of sample images. So if you ask it, for example, to generate a picture of a man holding an ice cream cone at sunset, it can.
The algorithms used in climate science are instead trained to generate pieces—hundreds or thousands of them—that can be plugged into bigger simulations to fill in the missing gaps. Just like DALL-E can conjure up images of apples when asked (because it has learned to identify them), the algorithms used in this research can generate models of processes important to climate modeling, such as droplet formation in clouds.
By putting simulated processes of that sort together with real-world data, researchers hope to create realistic predictions about Earth's rapidly changing climate, Schneider says.
"We can use the physical laws as far as we can, given the limited computational power at our disposal," he says. "What we cannot simulate, we can learn from data."