Caltech engineer exploits the repeating structure of turbulence to create a more complete model of the phenomenon
A Caltech engineer has unlocked some of the secrets behind turbulence, a much-studied but difficult-to-pin-down phenomenon that mixes fluids when they flow past a solid boundary.
Beverly McKeon, the Theodore von Kármán Professor of Aeronautics in the Division of Engineering and Applied Science, studies fluid mechanics. She specializes in turbulent flows, or technically speaking those with high Reynolds numbers. These types of flows are often seen in pipes and around aircraft and are of keen interest, for example, to aerospace engineers.
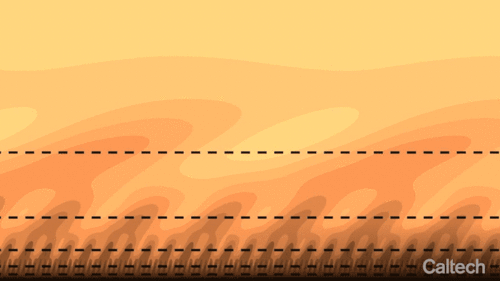
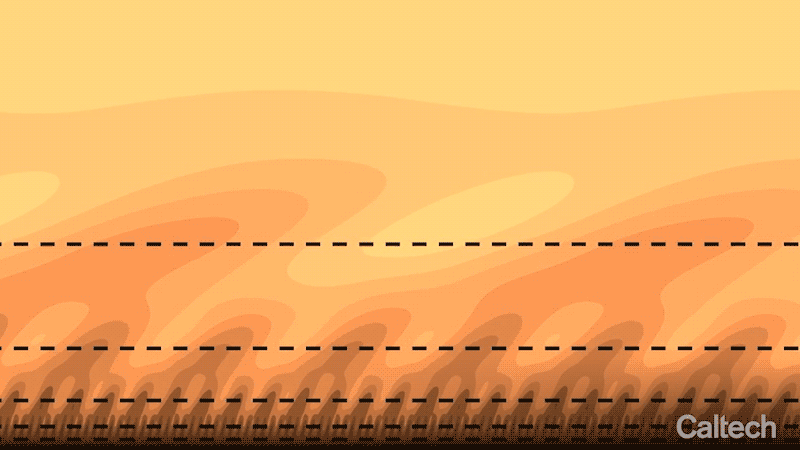
At the boundary where a fluid flows over a fixed structure, a turbulent boundary layer is created where the fluid interacts with the wall, creating eddies in the current. These eddies may seem to be random on first glance, but they actually create distinct patterns, with countless tiny eddies close to the wall; fewer but larger eddies located a little farther out; and even fewer, but still larger, eddies beyond those. These eddies have a significant impact on the fluid flow, helping to determine features such as its pressure, velocity, and density, which are important to understand when engineering an aircraft or industrial piping, for example.
In the 1950s and '60s, mathematician Alan Townsend of Cambridge University proposed that a lot of the important statistical properties of a turbulent flow could be described based on this concept of eddies as persistent, organized flow patterns that are, in essence, "attached" to a wall—even without a clear understanding of what those eddies actually are. Through the '80s and '90s, researchers led by Tony Perry, Ivan Marusic, and their colleagues at Australia's University of Melbourne built on Townsend's hypothesis to develop the "attached eddy" model of wall turbulence, which has proven to be effective at describing the statistical behavior of the common phenomenon.
The attached eddy model is an empirical representation of turbulence, obtained from quantifying actual features of the turbulence, and thus it is considered a "statistical" model. Engineers may also simulate turbulence with purely mathematical dynamic models, which use the equations of motion to describe the underlying physical dynamics in the system.
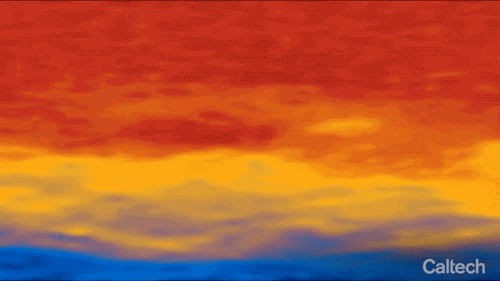
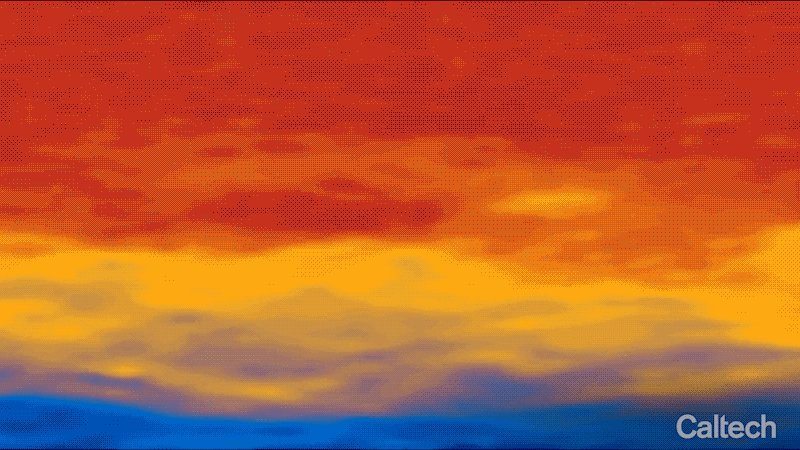
As an analogy, think of weather prediction. If you compiled 100 years' worth of weather reports, you could derive the average weather for an area and make a reasonable prediction about what the weather will be tomorrow. That is a statistical model. If you instead studied each of the physical systems that affect the weather—the ocean, the clouds, the topography—you could create a model that predicts the weather based on the various inputs to that system. That is a dynamical model.
A statistical model is easier to process, but a dynamical model is not a slave to the past; because it attempts to describe and understand what drives the system overall, it is capable of predicting future changes in the system that might be outside of the average norms. And like the weather, turbulence is a dynamic and ever-changing phenomenon.
The problem, however, is that simulating something as complex as turbulence using the equations of motion is an incredibly complex, computationally challenging task, McKeon says. Imagine trying to disassemble an entire car with just a monkey wrench. You might eventually get the job done, but it will take a lot of time and energy.
McKeon found a way to bridge the empirical and mathematical models by creating an equations-derived description of turbulence that exploits the fact that turbulence creates predictably repeating structures. The shape and structure of the eddies in turbulence are geometrically self-similar, meaning that each of the eddies are identical, just on different scales, similar to a fractal pattern.
Mathematically quantifying these repetitions, McKeon was able to formulate a dynamical model that describes turbulence using a sort of shorthand, allowing it to extrapolate how the overall system will look based on a zoomed-in look at just a few eddies. Because it describes an incredibly large-scale and complex system by boiling it down to a simple, repeating component, McKeon's model can generate mathematically useful models of turbulent systems using dramatically less compute power than was previously required.
"We knew that, underlying these very complicated structures, there had to be a very simple pattern. We just didn't know what that pattern was until now," says McKeon, who next plans to dig deeper into the model to quantify just how many eddies should be included to create an accurate representation of the whole.
The model could prove useful to engineers across industry who are looking to more easily simulate turbulent systems. But more importantly, it represents fundamental research that will help scientists and engineers better understand what drives those turbulent systems.
McKeon's study is titled "Self-similar hierarchies and attached eddies" and was published by Physical Review Fluids on August 26. Her work was funded by the Office of Naval Research.